일 | 월 | 화 | 수 | 목 | 금 | 토 |
---|---|---|---|---|---|---|
1 | 2 | 3 | 4 | 5 | ||
6 | 7 | 8 | 9 | 10 | 11 | 12 |
13 | 14 | 15 | 16 | 17 | 18 | 19 |
20 | 21 | 22 | 23 | 24 | 25 | 26 |
27 | 28 | 29 | 30 |
- BOXPLOT
- 부스트캠프 AI테크
- Numpy data I/O
- Python
- linalg
- Numpy
- type hints
- Operation function
- 최대가능도 추정법
- boolean & fancy index
- Python 특징
- 카테고리분포 MLE
- namedtuple
- Comparisons
- Python 유래
- 정규분포 MLE
- unstack
- python 문법
- seaborn
- pivot table
- 표집분포
- 가능도
- subplot
- Array operations
- ndarray
- VSCode
- dtype
- scatter
- 딥러닝
- groupby
- Today
- Total
또르르's 개발 Story
[38-2] Python 병렬 Processing 본문
Python에서 어떤 task를 병렬 처리로 실행하기 위해서는 Ray module을 사용합니다.
Ray module은 remote function을 사용해서 task들을 비동기적으로 처리할 수 있습니다.
1️⃣ 설정
Ray module을 설치해줍니다.
# install ray
!pip install ray
ray module을 init 해줍니다.
import ray
import time
# Check node
ray.init()
2021-03-17 06:26:32,365 INFO services.py:1174 -- View the Ray dashboard at http://127.0.0.1:8265
{'metrics_export_port': 59846,
'node_id': '5557bc90a7c69d388edae30f19ab624b9e2c0606055d5132f7c242e6',
'node_ip_address': '172.28.0.2',
'object_store_address': '/tmp/ray/session_2021-03-17_06-26-31_964138_1618/sockets/plasma_store',
'raylet_ip_address': '172.28.0.2',
'raylet_socket_name': '/tmp/ray/session_2021-03-17_06-26-31_964138_1618/sockets/raylet',
'redis_address': '172.28.0.2:6379',
'session_dir': '/tmp/ray/session_2021-03-17_06-26-31_964138_1618',
'webui_url': '127.0.0.1:8265'}
이때, ray module이 이미 init되어있다면 shutdown 함수를 사용해서 닫아주고, 다시 init을 실행합니다.
ray.shutdown()
2️⃣ ray 실행
remote function은 Ray의 프로세스에 의해 비동기적으로 실행됩니다.
따라서 아래의 코드는 ray에 의해서 비동기적으로 실행됩니다.
@ray.remote(num_cpus=1) # remote annotation을 사용해서 remote를 알림
def f(x):
time.sleep(1) # 1초 대기
return x
따라서 f(x)를 100번을 수행해 시간이 얼마나 걸리는지 확인합니다.
ray를 사용하면 4개의 task가 parallel하게 수행됩니다.
start = time.time()
# Start 4 tasks in parallel.
result_ids = []
for i in range(100):
result_ids.append(f.remote(i)) # remote를 통해 병렬로 만들어줌
# Wait for the tasks to complete and retrieve the results.
# With at least 4 cores, this will take 1 second.
results = ray.get(result_ids) # [0, 1, 2, 3]
>>> print("Ray 소요시간: {}".format(time.time() - start))
Ray 소요시간: 50.18559432029724
만약 sequential하게 실행하게 되면 100초가 걸리는 것을 알 수 있습니다.
def f(x):
time.sleep(1)
return x
start = time.time()
result_ids = []
for i in range(100):
result_ids.append(f(i))
>>> print("소요시간 : {}".format(time.time() - start))
소요시간 : 100.09278655052185
3️⃣ Task Dependency
한 Task가 끝나야 다른 Task를 실행할 수 있는 경우가 있습니다. 이런 경우를 Task Dependency가 있다고 합니다.
Ray로 코드를 돌렸을 때와 일반 sequencial 하게 코드를 돌렸을 때 비슷한 시간이 걸린 것을 알 수 있습니다.
(한 task가 끝나야 다른 task가 수행되기 때문에 병렬로 처리할 수 없습니다.)
Ray로 (depency한) task를 수행했을 경우입니다.
import numpy as np
@ray.remote
def create_matrix(size):
return np.random.normal(size=size)
@ray.remote
def multiply_matrices(x, y):
return np.dot(x, y)
size = 7000 # check O(?) as # of input increases
# Get the results with ray.
start = time.time()
x_id = create_matrix.remote([size, size])
y_id = create_matrix.remote([size, size])
z_id = multiply_matrices.remote(x_id, y_id)
z = ray.get(z_id)
>>> print("z_id_with_ray : {}".format(time.time() - start))
z_id_with_ray : 25.099600076675415
일반 sequencial하게 (depency 한) task를 수행했을 경우입니다.
import numpy as np
def create_matrix(size):
return np.random.normal(size=size)
def multiply_matrices(x, y):
return np.dot(x, y)
size = 7000 # check O(?) as # of input increases
# Get the results with ray.
start = time.time()
x_id = create_matrix([size, size])
y_id = create_matrix([size, size])
z_id = multiply_matrices(x_id, y_id)
z = z_id
>>> print("z_id_with_ray : {}".format(time.time() - start))
z_id_with_ray : 26.08303737640381
4️⃣ Aggregation
따라서 Ray를 사용하기 위해서는 Task에 dependency 하게 연산을 짜지 않는 것이 중요합니다.
아래 사진은 Task에 dependency한 연산 방법 (왼쪽)과 병렬적으로 짜진 연산 방법(오른쪽)입니다.
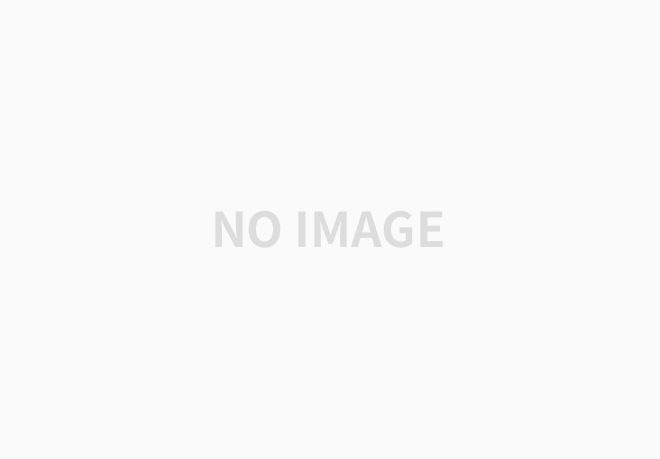
이 두 연산 방법을 코드로 작성하면 다음과 같이 속도 차이가 나는 것을 볼 수 있습니다.
import time
@ray.remote
def add(x, y):
time.sleep(1)
return x + y
start = time.time()
# Aggregate the values slowly. This approach takes O(n) where n is the
# number of values being aggregated. In this case, 7 seconds.
id1 = add.remote(1, 2)
id2 = add.remote(id1, 3)
id3 = add.remote(id2, 4)
id4 = add.remote(id3, 5)
id5 = add.remote(id4, 6)
id6 = add.remote(id5, 7)
id7 = add.remote(id6, 8)
result = ray.get(id7)
>>> print("Vanilla version : {}".format(time.time() - start))
start = time.time()
# Aggregate the values in a tree-structured pattern. This approach
# takes O(log(n)). In this case, 3 seconds.
id1 = add.remote(1, 2)
id2 = add.remote(3, 4)
id3 = add.remote(5, 6)
id4 = add.remote(7, 8)
id5 = add.remote(id1, id2)
id6 = add.remote(id3, id4)
id7 = add.remote(id5, id6)
result = ray.get(id7)
>>> print("Advanced version : {}".format(time.time() - start))
Vanilla version : 7.0304811000823975
Advanced version : 4.014824867248535
5️⃣ Reference
Modern Parallel and Distributed Python: A Quick Tutorial on Ray
Ray is an open source project for parallel and distributed Python.
towardsdatascience.com
'부스트캠프 AI 테크 U stage > 실습' 카테고리의 다른 글
[39-2] Quantization using PyTorch (0) | 2021.03.18 |
---|---|
[38-3] Pruning using PyTorch (0) | 2021.03.18 |
[37-2] PyTorch profiler (0) | 2021.03.17 |
[36-1] Model Conversion (0) | 2021.03.16 |
[34-4] Hourglass Network using PyTorch (0) | 2021.03.12 |