Notice
Recent Posts
Recent Comments
Link
일 | 월 | 화 | 수 | 목 | 금 | 토 |
---|---|---|---|---|---|---|
1 | 2 | 3 | 4 | 5 | ||
6 | 7 | 8 | 9 | 10 | 11 | 12 |
13 | 14 | 15 | 16 | 17 | 18 | 19 |
20 | 21 | 22 | 23 | 24 | 25 | 26 |
27 | 28 | 29 | 30 |
Tags
- 최대가능도 추정법
- 부스트캠프 AI테크
- Python 유래
- Numpy
- python 문법
- 카테고리분포 MLE
- seaborn
- groupby
- pivot table
- dtype
- Python
- namedtuple
- type hints
- ndarray
- subplot
- Numpy data I/O
- 표집분포
- unstack
- linalg
- 딥러닝
- 정규분포 MLE
- scatter
- boolean & fancy index
- VSCode
- 가능도
- BOXPLOT
- Operation function
- Comparisons
- Python 특징
- Array operations
Archives
- Today
- Total
또르르's 개발 Story
[32-2] Segmentation using PyTorch 본문
VGG-11 CNN 모델을 segmentation 문제를 풀기 위한 모델로 바꾸는 과정입니다.
1️⃣ VGG-11 BackBone
VGG-11의 기본 CNN 모델을 만듭니다.
BackBone은 Classification과 Segmentation을 구성하는 "뼈대" 모델을 의미하며, feature map을 추출하는 역할을 합니다.
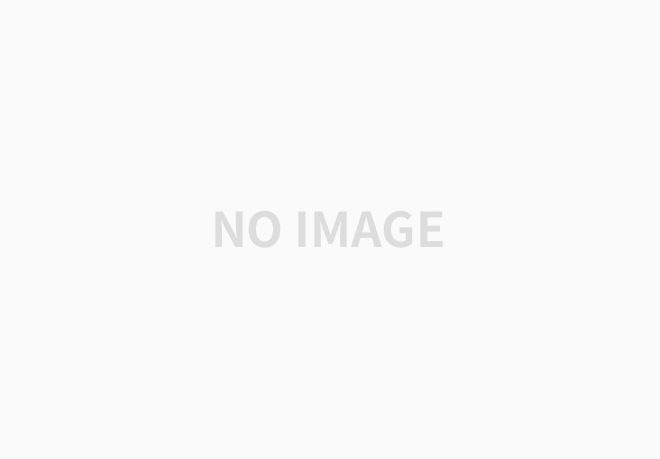
VGG11BackBone 코드는 다음과 같습니다.
import torch
import torch.nn as nn
class VGG11BackBone(nn.Module):
def __init__(self):
super(VGG11BackBone, self).__init__()
self.relu = nn.ReLU(inplace=True)
# Convolution Feature Extraction Part
self.conv1 = nn.Conv2d(3, 64, kernel_size=3, padding=1)
self.bn1 = nn.BatchNorm2d(64)
self.pool1 = nn.MaxPool2d(kernel_size=2, stride=2)
self.conv2 = nn.Conv2d(64, 128, kernel_size=3, padding=1)
self.bn2 = nn.BatchNorm2d(128)
self.pool2 = nn.MaxPool2d(kernel_size=2, stride=2)
self.conv3_1 = nn.Conv2d(128, 256, kernel_size=3, padding=1)
self.bn3_1 = nn.BatchNorm2d(256)
self.conv3_2 = nn.Conv2d(256, 256, kernel_size=3, padding=1)
self.bn3_2 = nn.BatchNorm2d(256)
self.pool3 = nn.MaxPool2d(kernel_size=2, stride=2)
self.conv4_1 = nn.Conv2d(256, 512, kernel_size=3, padding=1)
self.bn4_1 = nn.BatchNorm2d(512)
self.conv4_2 = nn.Conv2d(512, 512, kernel_size=3, padding=1)
self.bn4_2 = nn.BatchNorm2d(512)
self.pool4 = nn.MaxPool2d(kernel_size=2, stride=2)
self.conv5_1 = nn.Conv2d(512, 512, kernel_size=3, padding=1)
self.bn5_1 = nn.BatchNorm2d(512)
self.conv5_2 = nn.Conv2d(512, 512, kernel_size=3, padding=1)
self.bn5_2 = nn.BatchNorm2d(512)
def forward(self, x):
x = self.conv1(x)
x = self.bn1(x)
x = self.relu(x)
x = self.pool1(x)
x = self.conv2(x)
x = self.bn2(x)
x = self.relu(x)
x = self.pool2(x)
x = self.conv3_1(x)
x = self.bn3_1(x)
x = self.relu(x)
x = self.conv3_2(x)
x = self.bn3_2(x)
x = self.relu(x)
x = self.pool3(x)
x = self.conv4_1(x)
x = self.bn4_1(x)
x = self.relu(x)
x = self.conv4_2(x)
x = self.bn4_2(x)
x = self.relu(x)
x = self.pool4(x)
x = self.conv5_1(x)
x = self.bn5_1(x)
x = self.relu(x)
x = self.conv5_2(x)
x = self.bn5_2(x)
x = self.relu(x)
return x
2️⃣ VGG-11 Classification
classification은 물체의 분류를 담당하는 부분입니다.
class VGG11Classification(nn.Module):
def __init__(self, num_classes = 7):
super(VGG11Classification, self).__init__()
self.backbone = VGG11BackBone()
self.pool5 = nn.MaxPool2d(kernel_size=2, stride=2)
self.gap = nn.AdaptiveAvgPool2d(1)
self.fc_out = nn.Linear(512, num_classes)
def forward(self, x):
x = self.backbone(x)
x = self.pool5(x)
x = self.gap(x)
x = torch.flatten(x, 1)
x = self.fc_out(x)
return x
3️⃣ VGG-11 Segmentation
Segmentation은 img의 해상도를 줄여 물체의 위치를 파악하는 역할입니다.
여기서는 Fully Convolutional Networks를 사용해서 heatmap을 추출합니다.
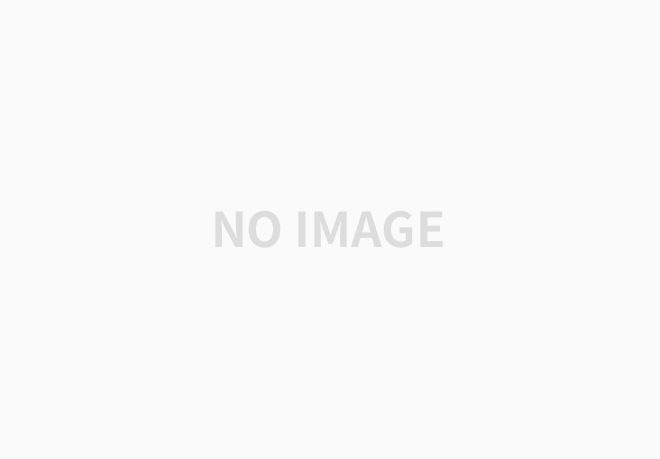
class VGG11Segmentation(nn.Module):
def __init__(self, num_classes = 7):
super(VGG11Segmentation, self).__init__()
self.backbone = VGG11BackBone()
# 512 -> 7로 FCN 실행, 1x1 Convolution 실행(이미 feature은 backbone에서 추출했기 때문에 channel만 줄여줌)
self.conv_out = torch.nn.Conv2d(512, num_classes, kernel_size=1)
self.upsample = torch.nn.Upsample(scale_factor=16, mode='bilinear', align_corners=False)
def forward(self, x):
x = self.backbone(x)
x = self.conv_out(x)
x = self.upsample(x)
return x
def copy_last_layer(self, fc_out):
# fc layer의 값을 FCN의 마지막 값으로 복사하는 함수
# fc_out은 Linear type
# fc_out.weight은 fc layer의 weight값이며, shape은 (7, 512)
# fc_out.weight.clone().detach().requires_grad_(True) : requires_grad option이 추가된 tensor 복사
fc_out_weight = fc_out.weight.clone().detach().requires_grad_(True)
conv_shape = self.conv_out.weight.shape # conv_out의 weight shape를 복사
self.conv_out.weight = torch.nn.Parameter(fc_out_weight.reshape(conv_shape)) # fc_out의 parameter를 self.conv_out에 복사, 이때, shape을 같게 만들어주어야함
return
4️⃣ Dataset 설정하기
DataSet을 새롭게 설정합니다.
# Dataset
import torch
from torchvision import transforms
from torch.utils.data import Dataset, DataLoader
import os
import cv2
import numpy as np
from glob import glob
class Dataset(Dataset):
def __init__(self, data_root, is_Train=True, input_size=224, transform=None):
super(Dataset, self).__init__()
self.img_list = self._load_img_list(data_root, is_Train)
self.len = len(self.img_list)
self.input_size = input_size
self.transform = transform
def __getitem__(self, index):
img_path = self.img_list[index]
# Image Loading
img = cv2.imread(img_path)
img = cv2.cvtColor(img, cv2.COLOR_BGR2RGB)
img = img/255.
if self.transform:
img = self.transform(img)
# Ground Truth
label = self._get_class_idx_from_img_name(img_path)
return img, label
def __len__(self):
return self.len
def _get_class_idx_from_img_name(self, img_path):
img_name = os.path.basename(img_path)
if 'normal' in img_name: return 0
elif 'test1' in img_name: return 1
elif 'test2' in img_name: return 2
elif 'test3' in img_name: return 3
elif 'test4' in img_name: return 4
elif 'test5' in img_name: return 5
elif 'incorrect test' in img_name: return 6
else:
raise ValueError("%s is not a valid filename. Please change the name of %s." % (img_name, img_path))
5️⃣ Loading model
Pretrained된 VGG-11 model을 load합니다.
## Model Loading
model_root = './model.pth'
modelC = VGG11Classification()
# torch.load(model_root) : orderdict로 구성되어 "layer이름 : tensor" 형태로 구성
modelC.load_state_dict(torch.load(model_root))
pretrained된 모델에서 "Fully Connected layer에 있는 weight"들을 "VGG11Segmentation의 FCL"에 옮겨야합니다.
## Copy Weight
modelS = VGG11Segmentation()
modelS.backbone = modelC.backbone # pre-trained된 VGG-11 backbone
w_fc = modelC.fc_out
modelS.copy_last_layer(w_fc) # FC에 있는 weight들을 FCL로 옮기는 과정
6️⃣ 시각화
Pre-trained된 Segmentation model에서 img와 label을 받아서 img에 segmentation이 표현됩니다.
import matplotlib
# Test on Segmentation
modelS.cuda().float()
modelS.eval()
for iter, (img, label) in enumerate(test_loader):
img = img.float().cuda() # img는 tensor
# Inference for Semantic Segmentation
res = modelS(img)[0] # modelS(img).shape = torch.Size([1, 7, 224, 224])
# res.shape = torch.Size([7, 224, 224])
heat = res[label[0]] # label = torch.Size([1])
# heat.shape = torch.Size([224, 224]) : 7개 class 중 label 번호의 224 x 224 가지고옴
resH = heat.cpu().detach().numpy()
heatR, heatC = np.where(resH > np.percentile(resH, 95)) # heatRow, heatColumn을 나눔 / np.where은 index를 반환 / np.percentile은 영상의 intensity 중 %를 구해주는 함수
seg = torch.argmax(res, dim=0) # dim=0으로 argmax를 구해 max의 index를 구함, 즉, 7개의 class 중 가장 큰 확률의 label을 뽑아옴
seg = seg.cpu().detach().numpy()
[segR, segC] = np.where(seg == np.int(label[0].cpu())) # seg와 label의 값이 같은 것들의 index를 segR, segC에 저장
# segR : [ 26 26 26 ... 214 214 214], segC : [132 133 134 ... 158 159 160]
resS = np.zeros((224,224))
for i, r in enumerate(heatR):
c = heatC[i]
if (r in segR) and (c in segC): # heatR == segR and heatC == segC일때
resS[r,c] = 1
want_to_check_heat_map_result = True # img에 segmentation 표시
# Plot segmentation result
matplotlib.pyplot.imshow(img[0].cpu().permute(1, 2, 0))
if want_to_check_heat_map_result:
matplotlib.pyplot.imshow(resH, cmap='jet', alpha=0.3) # 색깔 type : jet : 점들의 분포를 나타냄
matplotlib.pyplot.imshow(resS, alpha=0.4)
matplotlib.pyplot.show()
'부스트캠프 AI 테크 U stage > 실습' 카테고리의 다른 글
[34-3] CNN Visualization using VGG11 (0) | 2021.03.12 |
---|---|
[33-2] Pytorch Autograd (0) | 2021.03.11 |
[31-3] Scratch Training VS Fine Tuning (VGG-11) (0) | 2021.03.08 |
[31-2] VGG-11 구현 using PyTorch (0) | 2021.03.08 |
[25-1] GraphSAGE 모델 구현 using DGL Library (0) | 2021.02.27 |